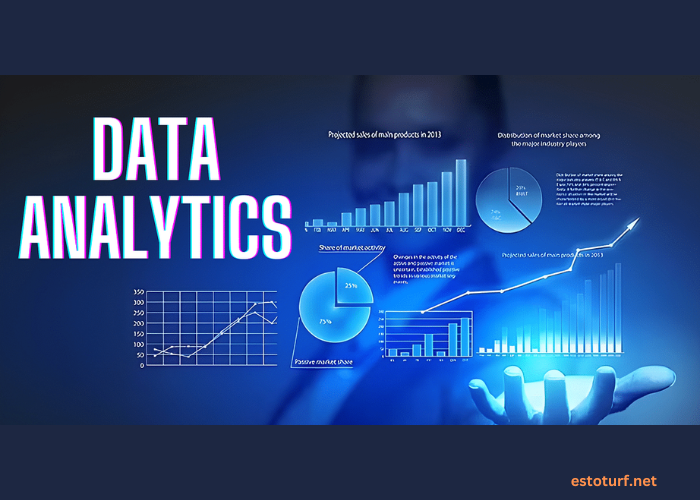
In today’s fast-paced digital landscape, data analytics plays a critical role in driving decisions and strategies across all sectors of business and technology. As we enter 2024, the evolution of technology continues to push the boundaries of what’s possible, shaping the future of data analytics in unprecedented ways. This article explores the most innovative data analysis techniques that are poised to redefine the analytics landscape in 2024, offering a glimpse into the future where data is not just valuable but the very currency of decision-making.
1. The Rise of Predictive Analytics
Predictive analytics has become a beacon of strategic foresight for businesses worldwide. By harnessing historical data and applying advanced algorithms and machine learning techniques, predictive analytics allows organizations to forecast future trends, behaviors, and events with remarkable accuracy. This shift towards anticipatory analysis is transforming how companies approach everything from marketing campaigns to inventory management and customer service. Real-world applications of predictive analytics can be seen across industries, including retail, finance, and supply chain management.
The significance of predictive analytics lies in its ability to shift decision-making from reactive to proactive. By predicting customer needs and market shifts, businesses can gain a competitive edge and lead the market. For example, in retail, predictive analytics is used to tailor product recommendations to individual customer preferences, enhancing the shopping experience and boosting sales. In the financial sector, it plays a crucial role in fraud detection, leveraging transaction data patterns to identify and prevent fraudulent activity before it occurs. The adoption and sophistication of predictive analytics are expected to increase in 2024, making it a critical tool for data-driven organizations.
2. The Evolution of Big Data Processing
The concept of “big data” has become central to strategic business planning. However, the sheer volume, velocity, and variety of data generated today pose significant challenges in processing and analysis. In 2024, remarkable advancements in technologies and methodologies address these challenges, enabling insights that were previously unattainable.
The evolution of big data processing is characterized by innovative solutions that enhance the efficiency and effectiveness of handling massive datasets. Platforms like Apache Hadoop and Spark have matured, offering scalable, distributed computing environments capable of processing petabytes of data at unprecedented speeds. These platforms distribute the processing load across clusters of computers, reducing analysis time from hours to minutes or even seconds.
Experience the game-changing advancements in big data processing with cloud-based analytics platforms. Discover scalable, on-demand resources that allow businesses to adapt their data processing capabilities effortlessly to meet their current needs, eliminating the need for substantial infrastructure investments. Seamlessly integrating with various data sources and applications, these platforms pave the way for more comprehensive analytics, enhancing the accuracy of insights derived from big data. Embrace this evolution in data processing for a more agile, efficient, and insightful analysis. Click here to explore the future of analytics in gambling, betting, and slots.
3. Emphasis on Data Privacy and Security
In the digital era, data privacy and security have become paramount concerns. High-profile data breaches have highlighted the devastating consequences of inadequate data security, ranging from financial loss to damaged reputations. These incidents have prompted stricter data protection regulations worldwide, such as the General Data Protection Regulation (GDPR) in Europe, which mandates rigorous data handling and privacy measures.
In response to these challenges, data analytics strategies are evolving to prioritize privacy and security. Techniques like differential privacy and homomorphic encryption are gaining traction. Differential privacy adds randomness to datasets, preventing the identification of individuals, while homomorphic encryption allows data to be processed in its encrypted form. These approaches enable businesses to leverage the power of data analytics while safeguarding personal information and complying with regulatory requirements.
Legislation plays a pivotal role in shaping the landscape of data privacy and security. Laws like GDPR enforce compliance and encourage more secure data analytics practices. Businesses are adopting a privacy-by-design approach, considering data protection at every stage of the analytics process. The emphasis on data privacy and security in 2024 reflects the balance between harnessing the power of data analytics and protecting individual rights. Finding innovative solutions to these challenges is crucial for building trust and credibility in the digital age.
4. AI and Machine Learning Integration
The integration of Artificial Intelligence (AI) and Machine Learning (ML) with data analytics has become a fundamental component of advanced analytics strategies. This fusion is driving the evolution of data analytics from basic descriptive analytics to more complex, predictive, and prescriptive analytics. AI and ML algorithms excel at identifying patterns and correlations in vast datasets, enhancing data analytics by providing more accurate forecasts, identifying emerging trends, and recommending actions.
AI-driven analytics platforms have emerged, offering powerful tools for data scientists and business analysts. These platforms automate data preparation and analysis, reducing the time and expertise required to derive insights. They clean, categorize, and analyze data, presenting findings in an accessible and actionable format. This democratization of data analytics allows a broader range of business users to leverage data in their decision-making processes, fostering a more data-driven culture within organizations.
The integration of AI and ML into data analytics enhances efficiency by automating routine data analysis tasks, allowing data scientists and analysts to focus on more strategic activities. It improves the accuracy of insights derived from data, enabling more precise decision-making. Most importantly, it empowers businesses to anticipate changes and adapt swiftly, providing a competitive edge. As AI and ML technologies continue to evolve, they promise to unlock new opportunities for innovation and growth.
5. Real-time Data Analytics
Real-time data analytics has become a critical asset for businesses seeking to stay ahead in their respective industries. The demand for instant insights stems from the increasing speed at which businesses and markets operate. Real-time analytics allows businesses to monitor operational performance, customer interactions, and market trends as they happen, leading to more agile and responsive decision-making processes.
Technological advancements have made real-time data analytics more accessible and effective. Stream processing frameworks like Apache Kafka and Apache Flink process data streams in real time, providing immediate insights into current data flows. In-memory databases and cloud computing have reduced the latency in data processing, ensuring that data is analyzed and acted upon as soon as it is generated.
Real-time data analytics has a profound impact on business operations. In retail, it can track inventory levels and customer demand simultaneously, triggering automatic restocking orders to prevent stockouts. In online services, it can detect and mitigate cybersecurity threats instantly, protecting both the business and its customers. Real-time insights into customer behavior enable personalized marketing and service delivery, enhancing customer satisfaction and loyalty. As technology continues to advance, the possibilities for real-time analytics will expand, further embedding its importance in data-driven decision-making.
6. The Future of Data Visualization
The future of data visualization is evolving to meet the increasing demand for accessible, intuitive, and interactive representations of complex datasets. Data visualization tools are becoming more sophisticated, offering a wider array of visualization types and interactive elements. These advancements make it easier for users to explore data, understand trends, and identify patterns. Dynamic dashboards allow users to drill down into specific data points, adjust parameters, and view the impact of different scenarios in real time.
Innovative tools and platforms leverage AI and machine learning to automate the creation of visualizations. These technologies suggest the most effective ways to present data based on its characteristics, the user’s objectives, and historical interaction patterns. This automation speeds up the process of generating insights and ensures that visualizations are optimized for comprehension and impact.
An essential component of the future of data visualization is storytelling. Effective data visualizations tell a story, conveying not just the “what” but the “why” behind the data. This approach engages a broader audience, facilitates a deeper understanding of the insights presented, and drives more informed decision-making.
Conclusion
As we look ahead to 2024, these innovative data analysis techniques are set to revolutionize the analytics landscape. Predictive analytics, big data processing, data privacy and security, AI and machine learning integration, real-time data analytics, and the future of data visualization will shape how businesses harness the power of data to drive success. Embracing these trends will enable organizations to unlock valuable insights, gain a competitive edge, and navigate the complexities of a data-driven world.